Jan 22, 2007
BCI: Communication and Restoration of Movement in Paralysis
Brain-Computer-Interfaces(BCI): Communication and Restoration of Movement in Paralysis.
J Physiol. 2007 Jan 18;
Authors: Birbaumer N, Cohen LG
The review describes the status of brain-computer- or brain-machine-interface research. We focus on non-invasive brain-computer-interfaces (BCIs) and their clinical utility for direct brain communication in paralysis and motor restoration in stroke. A large gap between the promises of invasive animal and invasive human BCI-preparations and the clinical reality characterises the literature: while intact monkeys learn to execute more or less complex upper limb movements with spike patterns from motor brain regions alone without concomitant peripheral motor activity usually after extensive training, clinical applications in human diseases such as Amyotrophic Lateral Sclerosis and Paralysis from stroke or spinal cord lesions shows only limited success with the exception of verbal communication in paralysed and locked-in patients: BCIs based on electroencephalographic potentials or oscillations are ready to undergo large clinical studies and commercial production as an adjunct or a major assisted communication device for paralysed and locked-in patients. However, attempts to train completely locked-in patients with BCI-communication after entering the complete locked-in state with no remaining eye-movement failed. We propose that a lack of contingencies between goal directed thoughts and intentions may be at the heart of this problem. Experiments with chronically curarised rats support our hypothesis, operant conditioning and voluntary control of autonomic physiological functions turned out to be impossible in this preparation. In addition to assisted communication BCIs consisting of operant learning of EEG slow cortical potentials and sensorimotor rhythm was demonstrated to be successful in drug resistant focal epilepsy and attention deficit disorder. First studies of non-invasive BCIs using sensorimotor rhythm of the EEG and MEG in restoration of paralysed hand movements in chronic stroke and single cases of high spinal cord lesions show some promise but need extensive evaluation in well-controlled experiments. Invasive BMIs based on neuronal spike patterns, local field potentials or electrocorticogram may constitute the strategy of choice in severe cases of stroke and spinal cord paralysis. Future directions of BCI research should include the regulation of brain metabolism and blood flow and electrical and magnetic stimulation of the human brain (invasive and non-invasive). A series of studies using BOLD-response regulation with functional magnetic resonance imaging (fMRI) and near infrared spectroscopy demonstrated a tight correlation between voluntary changes in brain metabolism and behaviour.
00:07 Posted in Brain-computer interface | Permalink | Comments (0) | Tags: brain-computer interface
Jan 15, 2007
The University of Washington Neural Systems Lab have created a humanoid robot you can control with your thoughts.
Via Mind Hacks
Researchers at the University of Washington Neural Systems Lab have created a humanoid robot you can control with your thoughts via a EEG-based non-invasive brain-computer interface.
Link to Neural Systems Lab robot info page
20:52 Posted in Brain-computer interface | Permalink | Comments (0) | Tags: brain-computer interface, artificial intelligence, robotics
Dec 18, 2006
EMG and EOG artifacts in brain computer interface systems
EMG and EOG artifacts in brain computer interface systems: A survey.
Clin Neurophysiol. 2006 Dec 12;
Authors: Fatourechi M, Bashashati A, Ward RK, Birch GE
It is widely accepted in the brain computer interface (BCI) research community that neurological phenomena are the only source of control in any BCI system. Artifacts are undesirable signals that can interfere with neurological phenomena. They may change the characteristics of neurological phenomena or even be mistakenly used as the source of control in BCI systems. Electrooculography (EOG) and electromyography (EMG) artifacts are considered among the most important sources of physiological artifacts in BCI systems. Currently, however, there is no comprehensive review of EMG and EOG artifacts in BCI literature. This paper reviews EOG and EMG artifacts associated with BCI systems and the current methods for dealing with them. More than 250 refereed journal and conference papers are reviewed and categorized based on the type of neurological phenomenon used and the methods employed for handling EOG and EMG artifacts. This study reveals weaknesses in BCI studies related to reporting the methods of handling EMG and EOG artifacts. Most BCI papers do not report whether or not they have considered the presence of EMG and EOG artifacts in the brain signals. Only a small percentage of BCI papers report automated methods for rejection or removal of artifacts in their systems. As the lack of dealing with artifacts may result in the deterioration of the performance of a particular BCI system during practical applications, it is necessary to develop automatic methods to handle artifacts or to design BCI systems whose performance is robust to the presence of artifacts.
18:08 Posted in Brain-computer interface | Permalink | Comments (0) | Tags: brain-computer interface
Researchers demonstrate EEG control of humanoid robot
Via ScienceDaily
University of Washington researchers have developed a brain-computer interface that allows humans to control the actions of robots through commands generated by analysis of EEG signals
Link
17:32 Posted in AI & robotics, Brain-computer interface | Permalink | Comments (0) | Tags: brain-computer interface, robotics
Dec 15, 2006
Online control of a brain-computer interface using phase synchronization
Online control of a brain-computer interface using phase synchronization.
IEEE Trans Biomed Eng. 2006 Dec;53(12 Pt 1):2501-6
Authors: Brunner C, Scherer R, Graimann B, Supp G, Pfurtscheller G
Currently, almost all brain-computer interfaces (BCIs) ignore the relationship between phases of electroencephalographic signals detected from different recording sites (i.e., electrodes). The vast majority of BCI systems rely on feature vectors derived from e.g., bandpower or univariate adaptive autoregressive (AAR) parameters. However, ample evidence suggests that additional information is obtained by quantifying the relationship between signals of single electrodes, which might provide innovative features for future BCI systems. This paper investigates one method to extract the degree of phase synchronization between two electroencephalogram (EEG) signals by calculating the so-called phase locking value (PLV). In our offline study, several PLV-based features were acquired and the optimal feature set was selected for each subject individually by a feature selection algorithm. The online sessions with three trained subjects revealed that all subjects were able to control three mental states (motor imagery of left hand, right hand, and foot, respectively) with single-trial accuracies between 60% and 66.7% (33% would be expected by chance) throughout the whole session.
00:19 Posted in Brain-computer interface | Permalink | Comments (0) | Tags: brain-computer interface
Nov 29, 2006
Brain-computer interface technology as a tool to augment plasticity
Brain-computer interface technology as a tool to augment plasticity and outcomes for neurological rehabilitation.
J Physiol. 2006 Nov 16;
Authors: Dobkin BH
Brain-computer interfaces are a rehabilitation tool for tetraplegic patients that aim to improve quality of life by augmenting communication, control of the environment, and self-care. The neurobiology of both rehabilitation and BCI control depends upon learning to modify the efficacy of spared neural ensembles that represent movement, sensation, and cognition through progressive practice with feedback and reward. To serve patients, BCI systems must become safe, reliable, cosmetically acceptable, quickly mastered with minimal ongoing technical support, and highly accurate even in the face of mental distractions and the uncontrolled environment beyond a laboratory. BCI technologies may raise ethical concerns if their availability affects the decisions of patients who become locked-in with brain stem stroke or amyotrophic lateral sclerosis to be sustained with ventilator support. If BCI technology becomes flexible and affordable, volitional control of cortical signals could be employed for the rehabilitation of motor and cognitive impairments in hemiplegic or paraplegic patients by offering on-line feedback about cortical activity associated with mental practice, motor intention, and other neural recruitment strategies during progressive task-oriented practice. Clinical trials with measures of quality of life will be necessary to demonstrate the value of near-term and future BCI applications.
22:35 Posted in Brain-computer interface | Permalink | Comments (0) | Tags: brain-computer interface
Model train controlled via brain-machine interface
Re-blogged from Pink Tentacle
Hitachi has successfully tested a brain-machine interface that allows users to turn power switches on and off with their mind. Relying on optical topography, a neuroimaging technique that uses near-infrared light to map blood concentration in the brain, the system can recognize the changes in brain blood flow associated with mental activity and translate those changes into voltage signals for controlling external devices. In the experiments, test subjects were able to activate the power switch of a model train by performing mental arithmetic and reciting items from memory.
Link to Pink Tentacle post
22:24 Posted in Brain-computer interface | Permalink | Comments (0) | Tags: brain-computer interface
Nov 21, 2006
Brain computer interface covered by Nature
17:00 Posted in Brain-computer interface | Permalink | Comments (0) | Tags: brain-computer interface
Nov 11, 2006
BCI as a tool to induce neuroplasticity
Brain-computer interface technology as a tool to augment plasticity and outcomes for neurological rehabilitation.
J Physiol. 2006 Nov 9
Authors: Dobkin BH
Brain-computer interfaces are a rehabilitation tool for tetraplegic patients that aim to improve quality of life by augmenting communication, control of the environment, and self-care. The neurobiology of both rehabilitation and BCI control depends upon learning to modify the efficacy of spared neural ensembles that represent movement, sensation, and cognition through progressive practice with feedback and reward. To serve patients, BCI systems must become safe, reliable, cosmetically acceptable, quickly mastered with minimal ongoing technical support, and highly accurate even in the face of mental distractions and the uncontrolled environment beyond a laboratory. BCI technologies may raise ethical concerns if their availability affects the decisions of patients who become locked-in with brain stem stroke or amyotrophic lateral sclerosis to be sustained with ventilator support. If BCI technology becomes flexible and affordable, volitional control of cortical signals could be employed for the rehabilitation of motor and cognitive impairments in hemiplegic or paraplegic patients by offering on-line feedback about cortical activity associated with mental practice, motor intention, and other neural recruitment strategies during progressive task-oriented practice. Clinical trials with measures of quality of life will be necessary to demonstrate the value of near-term and future BCI applications.
16:03 Posted in Brain-computer interface, Mental practice & mental simulation | Permalink | Comments (0) | Tags: brain-computer interface
Nov 05, 2006
An extended EM algorithm for joint feature extraction and classification in BCI
An extended EM algorithm for joint feature extraction and classification in brain-computer interfaces.
Neural Comput. 2006 Nov;18(11):2730-61
Authors: Li Y, Guan C
For many electroencephalogram (EEG)-based brain-computer interfaces (BCIs), a tedious and time-consuming training process is needed to set parameters. In BCI Competition 2005, reducing the training process was explicitly proposed as a task. Furthermore, an effective BCI system needs to be adaptive to dynamic variations of brain signals; that is, its parameters need to be adjusted online. In this article, we introduce an extended expectation maximization (EM) algorithm, where the extraction and classification of common spatial pattern (CSP) features are performed jointly and iteratively. In each iteration, the training data set is updated using all or part of the test data and the labels predicted in the previous iteration. Based on the updated training data set, the CSP features are reextracted and classified using a standard EM algorithm. Since the training data set is updated frequently, the initial training data set can be small (semi-supervised case) or null (unsupervised case). During the above iterations, the parameters of the Bayes classifier and the CSP transformation matrix are also updated concurrently. In online situations, we can still run the training process to adjust the system parameters using unlabeled data while a subject is using the BCI system. The effectiveness of the algorithm depends on the robustness of CSP feature to noise and iteration convergence, which are discussed in this article. Our proposed approach has been applied to data set IVa of BCI Competition 2005. The data analysis results show that we can obtain satisfying prediction accuracy using our algorithm in the semisupervised and unsupervised cases. The convergence of the algorithm and robustness of CSP feature are also demonstrated in our data analysis.
21:54 Posted in Brain-computer interface | Permalink | Comments (0) | Tags: brain-computer interface
Neural internet: web surfing with brain potentials
Neural internet: web surfing with brain potentials for the completely paralyzed.
Neurorehabil Neural Repair. 2006 Dec;20(4):508-15
Authors: Karim AA, Hinterberger T, Richter J, Mellinger J, Neumann N, Flor H, Kübler A, Birbaumer N
Neural Internet is a new technological advancement in brain-computer interface research, which enables locked-in patients to operate a Web browser directly with their brain potentials. Neural Internet was successfully tested with a locked-in patient diagnosed with amyotrophic lateral sclerosis rendering him the first paralyzed person to surf the Internet solely by regulating his electrical brain activity. The functioning of Neural Internet and its clinical implications for motor-impaired patients are highlighted.
21:53 Posted in Brain-computer interface, Cybertherapy, Future interfaces | Permalink | Comments (0) | Tags: brain-computer interface
Nov 01, 2006
Combined optimization of spatial and temporal filters for improving BCI
Combined optimization of spatial and temporal filters for improving brain-computer interfacing.
IEEE Trans Biomed Eng. 2006 Nov;53(11):2274-81
Authors: Dornhege G, Blankertz B, Krauledat M, Losch F, Curio G, Müller KR
Brain-computer interface (BCI) systems create a novel communication channel from the brain to an output device by bypassing conventional motor output pathways of nerves and muscles. Therefore they could provide a new communication and control option for paralyzed patients. Modern BCI technology is essentially based on techniques for the classification of single-trial brain signals. Here we present a novel technique that allows the simultaneous optimization of a spatial and a spectral filter enhancing discriminability rates of multichannel EEG single-trials. The evaluation of 60 experiments involving 22 different subjects demonstrates the significant superiority of the proposed algorithm over to its classical counterpart: the median classification error rate was decreased by 11%. Apart from the enhanced classification, the spatial and/or the spectral filter that are determined by the algorithm can also be used for further analysis of the data, e.g., for source localization of the respective brain rhythms.
22:35 Posted in Brain-computer interface | Permalink | Comments (0) | Tags: brain-computer interface
Oct 28, 2006
Brain-machine interfaces: past, present and future
Brain-machine interfaces: past, present and future.
Trends Neurosci. 2006 Sep;29(9):536-46
Authors: Lebedev MA, Nicolelis MA
Since the original demonstration that electrical activity generated by ensembles of cortical neurons can be employed directly to control a robotic manipulator, research on brain-machine interfaces (BMIs) has experienced an impressive growth. Today BMIs designed for both experimental and clinical studies can translate raw neuronal signals into motor commands that reproduce arm reaching and hand grasping movements in artificial actuators. Clearly, these developments hold promise for the restoration of limb mobility in paralyzed subjects. However, as we review here, before this goal can be reached several bottlenecks have to be passed. These include designing a fully implantable biocompatible recording device, further developing real-time computational algorithms, introducing a method for providing the brain with sensory feedback from the actuators, and designing and building artificial prostheses that can be controlled directly by brain-derived signals. By reaching these milestones, future BMIs will be able to drive and control revolutionary prostheses that feel and act like the human arm.
18:29 Posted in Brain-computer interface | Permalink | Comments (0) | Tags: brain-computer interface
Oct 26, 2006
Brain Waves Drawing
Brain Waves Drawing: Live Performance by Hideki Nakazawa: Nov 4-5, 2006 at Fuchu Art Museum, Tokyo Supported by Nihon Kohden.
Not to Draw by Hand. To Draw by Brain: Artists usually draw pictures by hand with brushes or pencils. However, the activities of brains must be more important and essential than the ones of hands at the moment of creating art. Therefore, I decided to draw pictures with electrodes being set on my head through controlling the activities of my own brain. The curved lines so-called "brain waves" in medicine must be the "drawings" in the world of fine art, directly drawn by my brain without using hands.
21:34 Posted in Brain-computer interface, Cyberart | Permalink | Comments (0) | Tags: brain-computer interface, cyberart
Oct 13, 2006
NEURObotics
Via Mind Hacks
NEURObotics is a newly-opened exhibition at London's Science Museum focusing on how emerging medical technology could expand human intelligence.
Topics include brain-scan lie detectors, enhancing brain function with TMS (magnetic pulses) and brain-computer interfaces.
The exhibition is free and runs until April 2007.
Link to Science Museum NEURObotics website.
Link to list of exhibits.
Link to BBC News story on the exhibition.
20:03 Posted in Positive Technology events | Permalink | Comments (0) | Tags: brain-computer interface
Oct 11, 2006
EEG-based brain-computer interface
Electro-encephalogram based brain-computer interface: improved performance by mental practice and concentration skills.
Med Biol Eng Comput. 2006 Oct 7;
Authors: Mahmoudi B, Erfanian A
Mental imagination is the essential part of the most EEG-based communication systems. Thus, the quality of mental rehearsal, the degree of imagined effort, and mind controllability should have a major effect on the performance of electro-encephalogram (EEG) based brain-computer interface (BCI). It is now well established that mental practice using motor imagery improves motor skills. The effects of mental practice on motor skill learning are the result of practice on central motor programming. According to this view, it seems logical that mental practice should modify the neuronal activity in the primary sensorimotor areas and consequently change the performance of EEG-based BCI. For developing a practical BCI system, recognizing the resting state with eyes opened and the imagined voluntary movement is important. For this purpose, the mind should be able to focus on a single goal for a period of time, without deviation to another context. In this work, we are going to examine the role of mental practice and concentration skills on the EEG control during imaginative hand movements. The results show that the mental practice and concentration can generally improve the classification accuracy of the EEG patterns. It is found that mental training has a significant effect on the classification accuracy over the primary motor cortex and frontal area.
21:49 Posted in Brain-computer interface, Mental practice & mental simulation | Permalink | Comments (0) | Tags: brain-computer interface, mental practice, motor imagery
Sep 30, 2006
Utilizing Gamma Band to Improve Mental Task Based Brain-Computer Interface Design
Utilizing Gamma Band to Improve Mental Task Based Brain-Computer Interface Design
IEEE Transactions on Neural Systems and Rehabilitation Engineering, Volume 14, Issue 3, Sept. 2006 Page(s): 299 - 303
Palaniappan, R.
A common method for designing brain–computer Interface (BCI) is to use electroencephalogram (EEG) signals extracted during mental tasks. In these BCI designs, features from EEG such as power and asymmetry ratios from delta, theta, alpha, and beta bands have been used in classifying different mental tasks. In this paper, the performance of the mental task based BCI design is improved by using spectral power and asymmetry ratios from gamma (24–37 Hz) band in addition to the lower frequency bands. In the experimental study, EEG signals extracted during five mental tasks from four subjects were used. Elman neural network (ENN) trained by the resilient backpropagation algorithm was used to classify the power and asymmetry ratios from EEG into different combinations of two mental tasks. The results indicated that 1) the classification performance and training time of the BCI design were improved through the use of additional gamma band features; 2) classification performances were nearly invariant to the number of ENN hidden units or feature extraction method.
19:24 Posted in Neurotechnology & neuroinformatics | Permalink | Comments (0) | Tags: brain-computer interface
Brain-computer interfaces for control of neuroprostheses
Brain-computer interfaces for control of neuroprostheses: from synchronous to asynchronous mode of operation.
Biomed Tech (Berl). 2006;51(2):57-63
Authors: Müller-Putz GR, Scherer R, Pfurtscheller G, Rupp R
Transferring a brain-computer interface (BCI) from the laboratory environment into real world applications is directly related to the problem of identifying user intentions from brain signals without any additional information in real time. From the perspective of signal processing, the BCI has to have an uncued or asynchronous design. Based on the results of two clinical applications, where 'thought' control of neuroprostheses based on movement imagery in tetraplegic patients with a high spinal cord injury has been established, the general steps from a synchronous or cue-guided BCI to an internally driven asynchronous brain-switch are discussed. The future potential of BCI methods for various control purposes, especially for functional rehabilitation of tetraplegics using neuroprosthetics, is outlined.
19:21 Posted in Brain-computer interface, Neurotechnology & neuroinformatics | Permalink | Comments (0) | Tags: brain-computer interface
Jul 29, 2006
Brain-activity interpretation competition won by Italian researchers
Via Mind Hacks
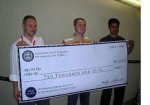
A team of three Italian researchers (Emanuele Olivetti, Diego Sona, and Sriharsha Veeramachaneni) won $10000 in a brain-activity interpretation competition. Entrants were provided with the fMRI data and behavioural reports recorded when four people watched two movies. The competitors' task was to create an algorithm that could use the viewers ongoing brain activity to predict what they were thinking and feeling as the film unfolded.
The Italian team resulted to be the most accurate, with a correlation of .86 for basic features, such as whether an instant of the film contained music. The full results are here.
20:55 Posted in Brain-computer interface, Neurotechnology & neuroinformatics, Persuasive technology | Permalink | Comments (0) | Tags: brain-computer interface
Jul 28, 2006
Brain-computer interfaces for 1-D and 2-D cursor control
IEEE Trans Neural Syst Rehabil Eng. 2006 Jun;14(2):225-9
Authors: Trejo LJ, Rosipal R, Matthews B
16:10 Posted in Brain-computer interface | Permalink | Comments (0) | Tags: brain-computer interface